Effective Ways to Find the Interquartile Range in 2025: Understand Key Steps!
The interquartile range (IQR) is a crucial statistical measure used to describe the spread of data points in a dataset. Understanding how to calculate the interquartile range can help illuminate the underlying patterns within data distribution. This article will guide you on the significance of IQR, how to find it step by step, and various methodologies employed for its calculation.
Understanding Interquartile Range
The interquartile range represents the range of the middle 50% of data points in a dataset. By calculating the IQR, analysts and researchers can evaluate the data variability and guard against the influence of outliers. The IQR provides insight into data spread and stability, making it essential for effective data analysis. In statistical terms, the IQR is computed by subtracting the lower quartile (Q1) from the upper quartile (Q3). Thus, the IQR formula can be expressed as:
IQR = Q3 - Q1
To elaborate, if your Q1 value is 5 and Q3 value is 15, the computation would be:
IQR = 15 - 5 = 10
In real-life applications, understanding the interquartile range can help in identifying risks in various fields, from finance to healthcare, where precise data interpretation is crucial.
Definition of Quartiles
To grasp the concept of the interquartile range, it’s vital to understand quartiles. Quartiles are data points that divide a dataset into four equal segments, providing a concise view of the distribution. The first quartile (Q1) marks the point below which 25% of data fall, while the third quartile (Q3) includes 75% of the data. Therefore, the middle 50% of data, depicted by IQR, is framed between Q1 and Q3. This framework is essential for grasping the breadth and central tendency in statistical analyses and is commonly illustrated in plots, such as a box and whisker plot, which visually represents each quartile along with outliers.
Importance of Interquartile Range
The interquartile range is vital for assessing data validity, primarily because it provides a robust measure less susceptible to outliers than range itself. In research, the IQR also serves as a means to analyze variances among different datasets, leading to better-informed decisions in areas such as finance, academia, and scientific fields. For instance, comparing datasets using IQR can help identify inconsistencies or shifts in data trends. A well-constructed statistical output using the IQR can highlight data variability, allowing professionals to address critical changes proactively.
Methods to Calculate Interquartile Range
Calculating the interquartile range involves several steps. There are multiple methods available to effectively compute IQR, from manual calculations based on ordered datasets to utilizing statistical tools. Here are some common methods:
Step-by-Step Interquartile Range Calculation
Finding the interquartile range through manual calculations is straightforward. First, organize your dataset in ascending order. Next, identify the quartile positions using the following approach:
- Calculate Q1, which is the value at position (n + 1)/4, where n denotes the total number of data points.
- Calculate Q3, located at position 3(n + 1)/4.
- Subtract Q1 from Q3 to obtain the IQR:
IQR = Q3 - Q1
For example, given an ordered dataset of [1, 3, 4, 6, 8, 9, 11, 15], Q1 would be the average of the 2nd and 3rd values (3 + 4)/2 = 3.5, while Q3 would be determined similarly, resulting as 11.
Using Statistical Tools for IQR Calculation
In today’s data-heavy environment, reliance on statistical software and tools is commonplace for IQR calculations. Programs like Excel, R, and Python provide streamlined functionalities to increase efficiency and accuracy. In Excel, you can employ the IQR function for quick calculations directly. A formula to extract quartiles can be defined as follows:
Q1 = QUARTILE.EXC(data_range, 1)
Q3 = QUARTILE.EXC(data_range, 3)
IQR = Q3 - Q1
Alternatively, in Python, libraries like Pandas can assist effortlessly in performing IQR analyses on large datasets, making life easier for data analysts.
Interquartile Range for Outliers Analysis
Another crucial application of the interquartile range is in outlier detection. Outliers are extreme values that lie significantly outside the interquartile range. Statistically, values beyond Q1 - 1.5 * IQR and Q3 + 1.5 * IQR are often categorically regarded as outliers. By employing IQR in this manner, data analysts can effectively isolate data points that may distort overall results or indicate erroneous entries, ensuring accurate analysis and interpretation.
Graphical Representations of IQR
Visualizing the interquartile range often aids in comprehending data spread and the presence of outliers. Techniques like the box plot graphically depict the data's central tendency and spread, allowing for instant pattern recognitions. The box plot effectively summarizes the quartiles, with the ends of the boxes representing Q1 and Q3, and a line in the box showcasing the median. Whiskers extend to the smallest and largest values in the dataset that are not considered outliers:

As shown in the image, the IQR is represented as the length of the box itself. This visualization not only enhances understanding but also promotes quick data interpretation in professional settings.
Key Takeaways
- The IQR is fundamental in assessing data variability and identifying outliers.
- Understanding how to find the interquartile range step by step enables precise data analyses.
- Utilizing statistical tools like Excel and Python facilitates effective IQR calculations.
- Visual tools such as the box plot reinforce the understanding of data distributions.
FAQ
1. What is the significance of the interquartile range in statistics?
The significance of the interquartile range lies in its power to convey data variability, while being less sensitive to outliers compared to the overall range. By focusing on the middle 50% of data, it provides a more robust measure of dispersion, helping in sound statistical analysis and decision-making.
2. How can I identify outliers using the IQR?
To identify outliers, first calculate the IQR by finding Q1 and Q3. Then, any data point that is less than Q1 - 1.5 * IQR or greater than Q3 + 1.5 * IQR is considered an outlier. This method allows researchers to focus on potential data inaccuracies while preserving analytical integrity.
3. Can I apply IQR analysis in real-life scenarios?
Absolutely! The interquartile range is leveraged across various fields - from finance to healthcare - to guide decision-making based on data insights. Its ability to highlight variability and potential outliers makes it invaluable in real-life applications.
4. How do I calculate quartiles using Excel?
Excel simplifies the calculation of quartiles using the QUARTILE function. You can input your data range and specify the quartile you wish to calculate. For example, use =QUARTILE.EXC(data_range, 1)
for Q1 and =QUARTILE.EXC(data_range, 3)
for Q3 to compute the IQR with simple arithmetic thereafter.
5. What resources can assist in understanding interquartile range better?
Several resources, such as statistical textbooks, online courses, and interactive data visualization tools, provide complex analyses for a better grasp of the interquartile range. Engage with platforms like Coursera and Khan Academy, or refer to online articles like those found on TipHack for structured learning pathways on statistical concepts.
By following these guidelines surrounding the interquartile range, and utilizing the methods discussed, anyone can effectively analyze and interpret data distributions in a meaningful way.
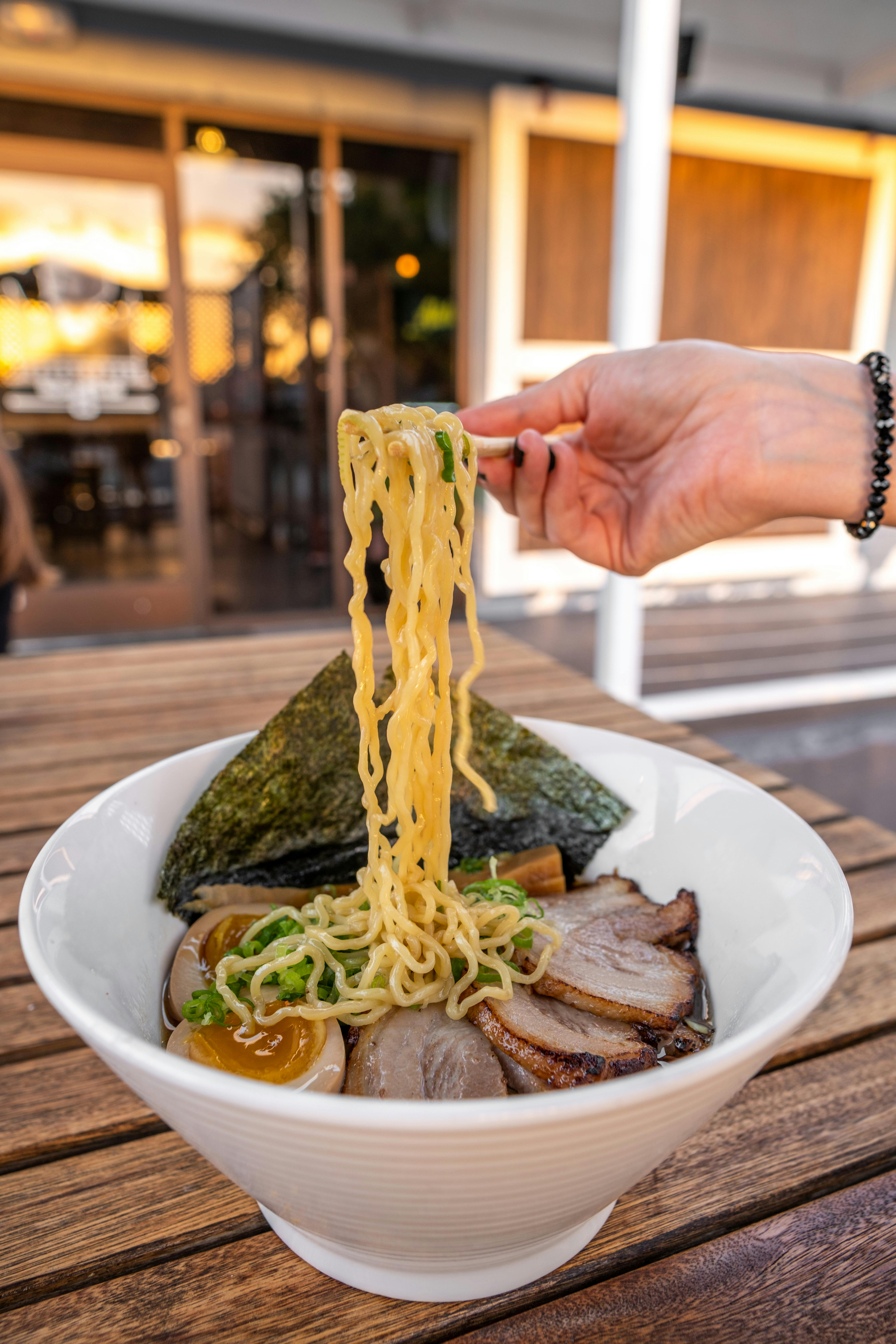